Happy New Year everyone!! Welcome to all the new subscribers that have joined us over the holiday period! If you haven’t already, you can read my last article on where crypto is headed here.
Stay up to date with Superfluid by subscribing here:
So the GPT-3 hype train is still in full swing, and over the Christmas and New Year break, we’ve seen more tools being released on a daily basis and numerous courses showing how anyone can use GPT-3 to automate the boring parts of their life.
Whilst it’s cool that we can now generate slide decks, stories and more with just a click of a button, in the grand scheme of things these are just small wins. One of the areas where AI can have a significant impact is in the field of drug discovery.
We all know that drug discovery is a slow and costly process, typically taking over 10 years and a billion dollars to bring a drug to market. And at the end of all that, only 10-20% of drugs are successful in going through clinical trials and receiving marketing approval.
But AI has the potential to significantly improve and accelerate this process, leading to the development of new treatments for various diseases more quickly and efficiently. This week, we dive deep into the drug discovery process and how the introduction of AI/ML has made a significant impact on the industry over the last decade.
What does it take to bring a drug to market?
To begin with, scientists need to identify a ‘target’ which will be a specific protein or genome that is associated with a particular disease or condition. This stage can take years of deep research by discovery biologists including strenuous amounts of literature review for insights into different genomes and molecular pathways.
Once a target is found, the target is then put through rigorous compound screening. Compounds are substances that have the potential to interact with the target protein/genome and either inhibit or stimulate its activity depending on the desired effect. Compound screening is largely an exercise of trial and error. Trying 1000s of compounds against a target in the hopes of having a few positive results.
If a promising compound(s) is identified this is then pushed through preclinical studies involving lab and animal testing to define toxicity, absorption rates and efficacy of the chosen compounds. If successful, the compound gets through to clinical trials which are a three-phase multi-year process of testing the drug on humans. Typically only 5-10% of drugs that start clinical trial testing pass through successfully. Tbh, this basically sounds like VC investing…..
So where does AI/ML fit into all of this?
Target Identification
Right at the start of the drug discovery process, target identification is a very arduous process with the potential for high failure rates during testing. As a result, AI applications for target identification can:
Significantly reduce the amount of menial work required to find targets to test by processing large amounts of academic research and data
Identify targets based on prior drug developments and research that may not be immediately obvious.
In line with no. 2, BenevolentAI uses ‘knowledge graphs’, which represent the relationships between targets, inhibitors and diseases as interconnected nodes. By studying known relationships, scientists are more likely to identify new or unexpected relationships. E.g., certain molecules might inhibit the same subset of proteins but are used to treat different diseases. As a result, there is a stronger likelihood that these molecules might be able to be used interchangeably to treat other adjacent diseases.
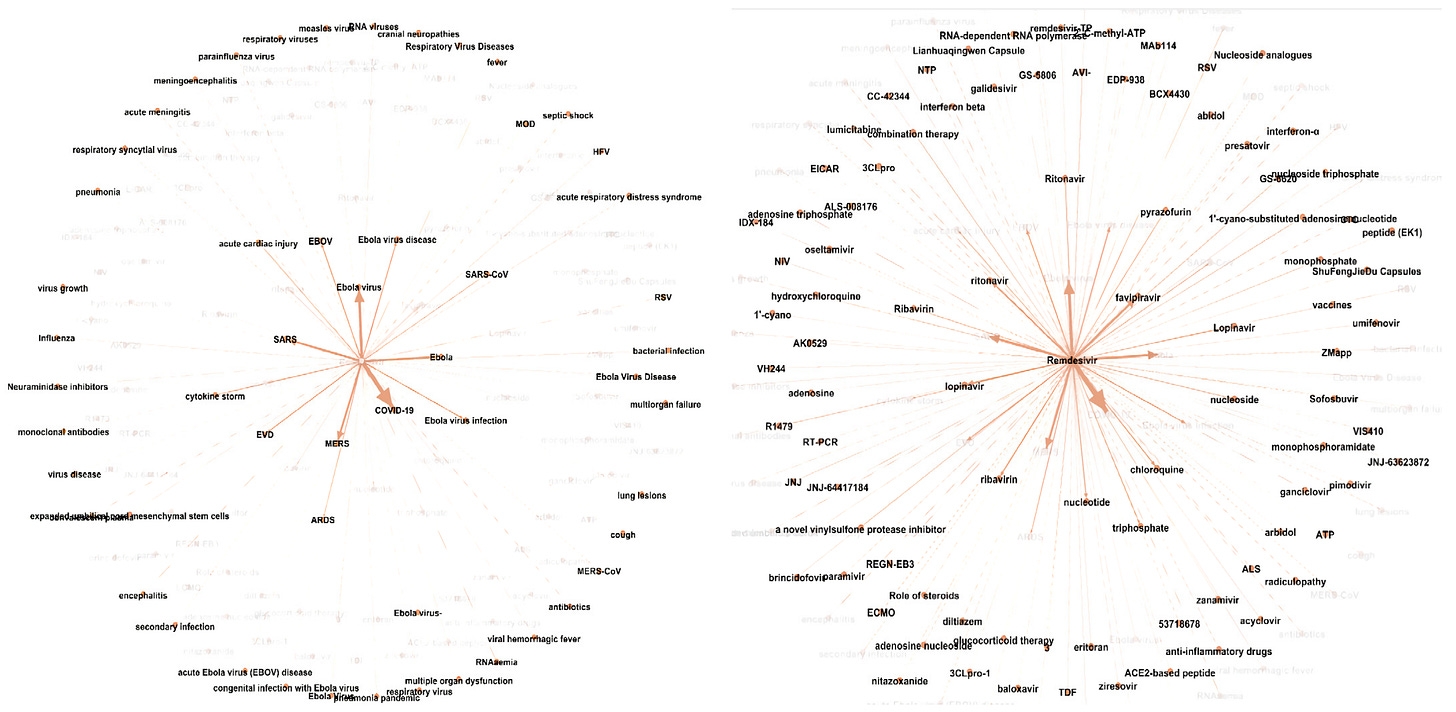
For example, in 2020, at the peak of the pandemic, BenevolentAI used AI to search through a knowledge graph built around viral infections and inflammatory responses. From this, they were able to identify Baricitinib, a drug approved to treat rheumatoid arthritis as the strongest candidate to treat COVID patients. Subsequently, the USA first authorised use of baricitinib in November 2020, Japan in April 2021, India in May 2021, the UK in May 2022 showing how quickly the drug was pushed through multiple rounds of clinical trials and into the market. This all began with a rapid data scanning and mining process, with the entire knowledge graph scan process taking under 90 minutes to complete. Can you imagine how long it would’ve taken for a group of 5 scientists to sift through Hard Drives full of research reports to get to the same result???
It pays heavily to identify molecules that will fail ASAP, as it means that scientists can take a heavy iteration-focused approach toward drug discovery, rather than a laborious research-heavy approach. AI/ML can be used to tentatively vet drugs for a certain threshold of efficacy prior to being put through the rigorous clinical trials process. By doing this, time and $$$ can be saved.
Compound Screening
Trying to screen 1000s of chemical compounds against a drug target is also a long process if carried out physically in a laboratory. Instead, ML tools have been created to undertake this process virtually. Deep Learning algorithms can ingest sophisticated chemistry and physics principles and can make reasonably accurate predictions on the efficacy of a drug and its ability to bind to a target, as well as undertaking Quantitative Structure-Property Relationship (QSPR) and Quantitative Structure-Activity Relationship (QSAR).
QSPR models predict the properties of a chemical compound based on its molecular structure. For example, a QSPR model can be used to predict the solubility, melting point, or boiling point of a compound. The molecular structure of a compound is related to its properties and by understanding this relationship, we can predict the properties of new compounds before they are synthesized.
QSAR models predict the biological activity of a chemical compound based on its molecular structure. These models can be used to predict the toxicity, efficacy, or other pharmacological properties of a compound. The molecular structure of a compound is related to its activity and by understanding this relationship, we can predict the activity of new compounds before they are tested in vivo.
Both of these types of analysis are crucial for tightening the funnel on what drugs actually get through to clinical trials vs what goes back to the drawing board. Again, early identification of drugs that have potential flaws in them will save millions later on.
Novel Drug Design
Generative modelling has been used to design small-molecule drugs and to predict their efficacy in treating a given condition. In most cases, generative modelling tends to be used to explore new chemical spaces with a heavy focus on the novelty of generated chemical structures.
For example, researchers from Northwestern University used a deep generative model which optimises the synthetic feasibility of a compound, its effectiveness against a given biological target and its novelness compared to other molecules in the literature and patent space.
To do this, the researchers created a two-step model that combined a few techniques including reinforcement learning, variational inference and tensor decompositions. To begin with, the model was trained on a data set of chemical compounds mapped to a continuous space of 50 dimensions. Typically chemical compounds are represented in a discrete form (pictured below), however, in this case, the continuous representation allows the algorithm to identify patterns in the data that would be difficult to create using a discrete molecular graph.
As the second step, the algorithm employs reinforcement learning to discover new chemical compounds. Reinforcement learning uses reward functions that guide the algorithm's future results and decision-making. In this case, the researchers used 3 different self-organising maps (a form of a neural network) as reward functions, with 1 of these specifically optimising for the novelty of a compound.
Overall, they obtained an initial output of 30k chemical structures, which were filtered down to a random set of 40 structures. 39 of these were likely to fall outside the scope of any published patents, and 6 were chosen for further experimental validation, with 1 being tested on mice. All of this occurred in just 46 days, a significant time improvement from the numerous months and years that it traditionally takes.
Where to from here?
All of that sounds nice and it looks like there’s been strong progress made in terms of actually getting AI-enabled drugs to market, what else is there to achieve?
Well, we’re really only at the tip of the iceberg in terms of the amount of progress made, and how much is left to uncover in the deep black box that is AI x BioTech.
As with anything AI/ML related, a rich dataset is crucial for success. Whilst public datasets do exist such as DrugBank which has over 500k drugs, and the Protein Data Bank which has over 160k 3D models of biological molecules, the data quality of this data is questionable and unstructured. At the moment, startups need to do extensive data engineering and potentially even enter unsavoury contracts with Big Pharma companies in the hopes of accessing high-quality proprietary data. Moreover, biology is heavily context-dependent. Tech teams dealing with large amounts of clinical and chemical data that is unstructured is a disaster waiting to happen.
Whilst I’ve largely focused on the impact of AI on the scientific side of drug design, there are so many other parts of the value chain where AI can play a significant role. Areas such as logistics for clinical trials, patient matching and engagement, as well as running diagnostics and claims processing are all crucial parts of getting a drug to market. My largely uninformed opinion is that these ‘boring’ areas of drug discovery are likely to be higher value than taking pot shots on goal at trying to find the next big drug using AI. If you’re building in this space, please do reach out to me on Twitter or Linkedin!
This was one of my first forays into biotech and drug discovery! I’m thinking of writing a follow-up piece to this one if people are interested - please let me know what you think in the comments. There’s so much to explore and learn!
Make sure to subscribe now to not miss the next article.
How did you like today’s article? Your feedback helps me make this amazing.
Thanks for reading and see you next time!
Abhi